What really is the Deep Space Construction Intelligence Platform?? For the past 5 years or so I have been diving deep into Construction data and automation, and along with Michael Clothier we used our decades of BIM and VDC experience to build Deep Space. Check out this video to find out what Deep Space actually is… and let us know what you think in the comments!
Category: Deep Space
I came across an interesting issue today. We were automating Revit data parameters for a mandated IFC deliverable, but we came across some Shared Parameters that were added incorrectly (wrong Parameter Name for the Shared Parameter GUID). Ok… let’s just remove them from Project Parameters, right?
Seems nice and easy. Now let’s add the ‘right’ Shared Parameter using Shared Parameters dialog box. Uh oh! Revit still remembers the OLD Parameter Name. Not good. This issue was well described by Jay Merlan over here. From his post:
“If you want to test this limitation, try deleting a Shared Parameter from a Revit model and loading a parameter with the same GUID but a different name. It will always assume the original parameter name that was defined in the project or family.“
Ok, now what? We had already acquired all the data into Deep Space, so we could open up the Data App. From here, we can search for either the Shared Parameter GUID or the ‘wrong’ parameter name from the latest data capture.
Now for the cool part – the ParameterId that Deep Space has captured is actually a Revit Element ID. We can copy the value from Deep Space, use Select by Id in Revit, then delete the Parameter PROPERLY. This is the way to truly get rid of a Shared Parameter from your model. We aren’t really worried about losing data, because all historical parameter data is captured into Deep Space anyway!
These are the key points:
- Removing a Shared Parameter from Revit interface doesn’t really delete it
- Deep Space ParameterId for that shared parameter is ACTUALLY an Element ID
- We can use that to select the Parameter “Element” in Revit and delete it properly! (use Delete key or the little red X on ribbon)
The total workflow we used on this particular project was:
- Setup Deep Space
- Sync Data from Revit
- Configure and use LOI Report app in Deep Space – this tells us which Shared Parameters have incorrect GUIDs
- Now use Data app in Deep Space to query and search for Parameter info. Use this to fix the model Shared Parameters
- Use Deep Space Write Back functionality to write data captured in a Deep Space web input portal straight back into the model
- Configure some IFC Export settings (category mapping and attribute settings) then export the ultimate IFC deliverable for the client.
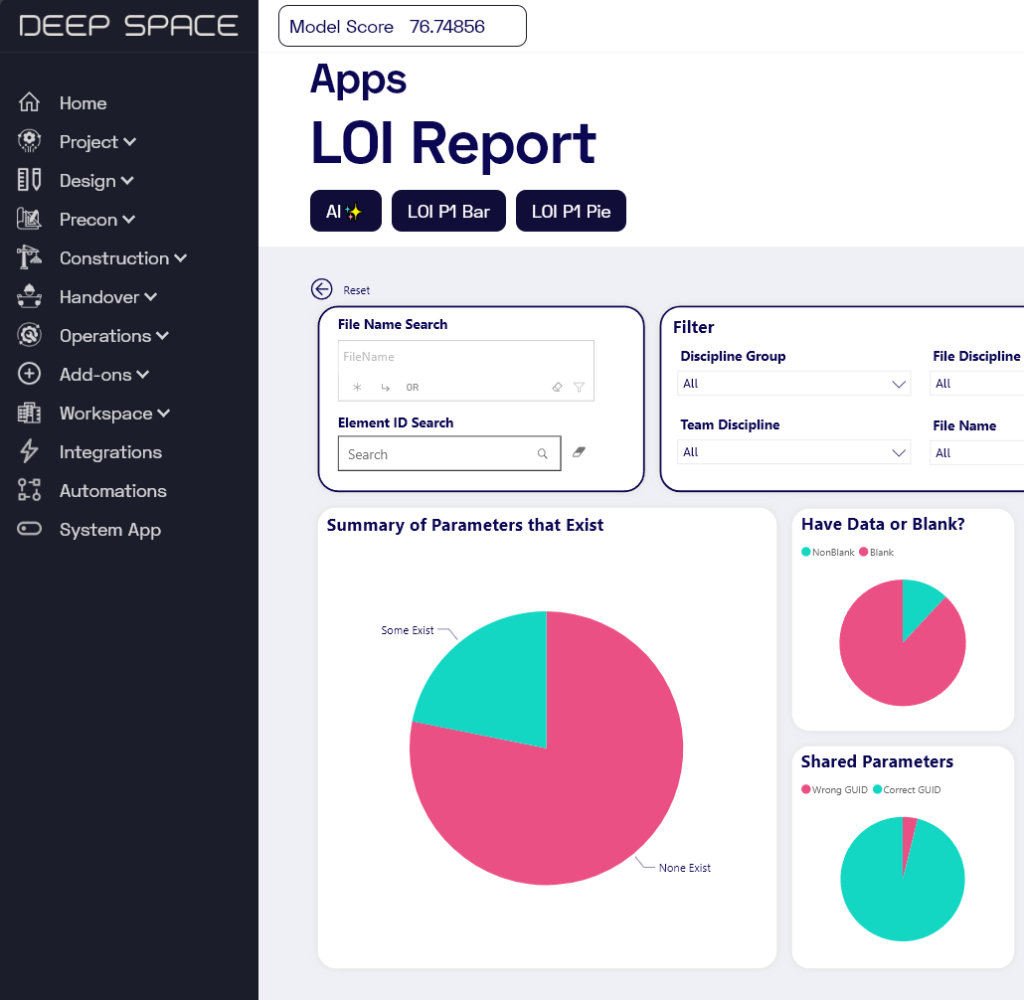
Around 9 months ago I decided to step back from the majority of my consulting work and focus on Deep Space, a digital construction project intelligence platform built by myself and Michael Clothier. After a lot of learning, pitching, refining, and due diligence, we were extremely pleased to reach a milestone last week. We were able to finally share with the world that Deep Space has been officially funded by VentureOn Partners, a forward-thinking ConTech + PropTech SaaS investment company in Australia!
Here is part of the press release:
VentureOn Partners Pty Ltd (VentureOn), a leading venture capital firm headquartered in Brisbane, Australia, is thrilled to announce a significant investment in Deep Space, an innovative construction technology (contech) company. This strategic investment marks a pivotal moment in the contech journey.
Luke Johnson, Co-Founder and CEO of Deep Space, expressed his enthusiasm about the partnership: “We are delighted to welcome VentureOn as a strategic investor,” said Johnson. “Their commitment and operational collaboration will undoubtedly propel Deep Space to new heights in the construction technology landscape.”
“VentureOn’s investment is a testament to the value our solutions bring to major construction projects globally,” said Michael Clothier, Co-Founder and COO of Deep Space. “This partnership aligns perfectly with our vision of expanding our reach and impact.”
Today, Deep Space is trusted by industry professionals globally and has proven instrumental in enhancing the efficiency of major construction projects. Deep Space’s unique solutions have already demonstrated their value in high-profile projects such as the Hong Kong Airport and Cross River Rail, significantly improving their efficiency and data handling capabilities. Their suite of services, including data acquisition add-ins, secure cloud storage, and pioneering Core Thread Technology, truly sets them apart in the market.
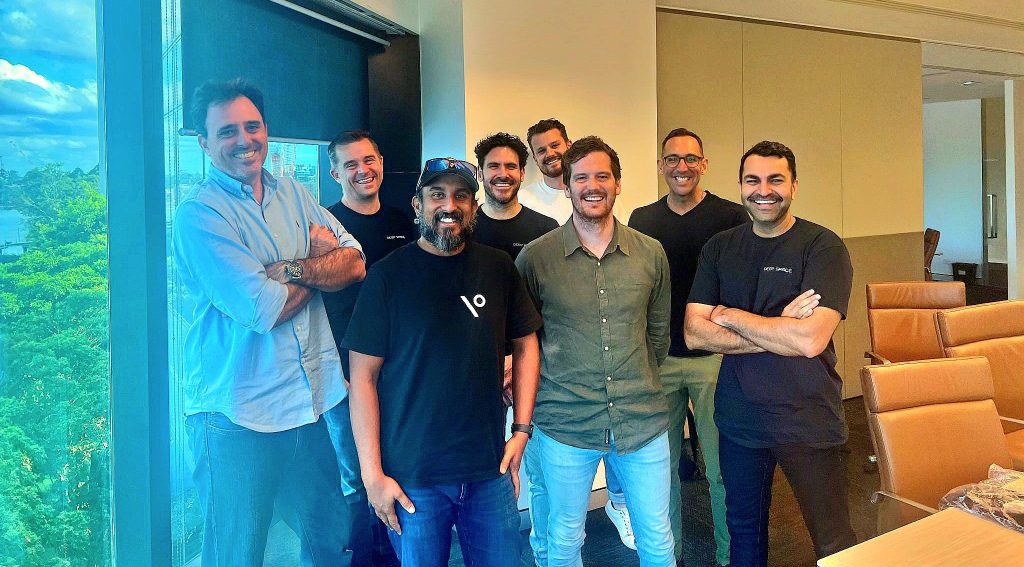
VentureOn’s investment comes with a clear vision of working closely with Deep Space to expand its operations both domestically and scale internationally. This partnership is set to drive growth and development, with a focus on scaling the company and achieving a Series A investment for continued global expansion.
“This investment reaffirms VentureOn’s vision of nurturing and scaling local SaaS companies to achieve international success,” said Sean Diljore, Co-Founder and General Partner at VentureOn. “Our hands-on approach to growth and strategy makes us a valuable partner for companies like Deep Space.”
VentureOn’s commitment to the construction tech and property tech domains is evident through this strategic investment, which comes shortly after the acquisition of Story Bridge Ventures and PropTech BNE earlier in October. The company believes in the transformative potential of these domains and aims to support innovations that enhance the construction and property industry.
This new operational collaboration forms a vital foundation for the partnership with Deep Space.
“We are enthusiastic about the vast potential of Deep Space,” said Ricky Sevta, Co-Founder and General Partner at VentureOn. “Through VentureOn’s investment and operational support, our goal is to expedite Deep Space’s growth and broaden its global presence, delivering innovative solutions to the construction domain.”
Read the rest of the press release here.
Follow Deep Space here.
Follow VentureOn Partners here.
Yes, I’m going to do this. I’m going to write another post about a widely used term and try to untangle reality from the fiction. Let’s talk about the term “AI”… Mr Gates wrote a compelling article recently about AI Agents specifically, and even Mr Clippy got a slightly dishonourable mention. But hold on to your chatbots hats, because we are going to go right back to basics…
First, let’s define some terms related to AI.
Intelligence
The faculty of understanding; intellect.
Artificial
made or constructed by human skill, especially in imitation of, or as a substitute for, something which is made or occurs naturally;
Now, we might assume that Artificial Intelligence means something man-made that has the faculty of understanding, right? Right?
As a verb, to understand means to know or realise the meaning of words, a language, what somebody says, etc.
Now, let’s compare this with a common definition of artificial intelligence…
the study and development of computer systems that can copy intelligent human behaviour.
Or, on Wikipedia:
Artificial intelligence (AI) is the intelligence of machines or software, as opposed to the intelligence of humans or animals.
And finally, AGI:
An artificial general intelligence (AGI) is a hypothetical type of intelligent agent. If realised, an AGI could learn to accomplish any intellectual task that human beings or animals can perform.
Now, you may ask, “what’s with all the definitions?” My personal feeling is that the perception of commonly available AI tech progress is largely overestimated and misunderstood, and that the ultimate aspirations of AGI are some distance away. So what’s with all the AI hype?
A few things definitely changed in the past couple of years. Tools claiming to be AI became widespread, and the technology started to be viewed as useful and cool. Let’s start with OpenAI and ChatGPT. It took the world by storm! It has a massive budget and huge backing. But what is it really?
ChatGPT, PaLM / Bard, and LLaMa are all Large Learning Models. They consume massive amounts of data, build a kind of neural network, and then you can converse with them. But guess what? They don’t have the human faculty of understanding. They are effectively guessing what the next word should be based on context and a massive set of data and processing power. Almost all Copilot-like technology works on this basis, except you can introduce your own contextual data into the model. LLMs must be trained on data that was ultimately produced by humans when in its “most raw form.”
Is it cool? Yes. Is it useful? Sure. Can it save you time? Yep. Is it anywhere near human intelligence? Of course not. You can’t measure a faculty of true understanding based on outputs and interactions. Understanding happens on the inside. I don’t believe I’m saying anything too controversial, but I do believe we should occasionally ask the question “what level of AI tech do we really have right now?”
What about image generation, specifically text-to-image models like DALL-E 3, Imagen, and Midjourney? These actually use some variation of an LLM – the input text is converted and fed to a generative image model. That model has been trained on massive amounts of text and image data scraped from all over the place. It seems creative but isn’t it really a tool that adapts and morphs known images into some new variation? Again, they must be trained on data that was ultimately produced by humans when in its “most raw form.”
Perhaps I’m oversimplifying, but does the current global state of AI tech approach anywhere near the creativity and original ingenuity of a human? Not even close. They are powerful tools that are transformative and disruptive. But they are really Super Guess Makers. They produce contextual linear things that ultimately are a version of “the system knows billions of types of representational data, and it can try to build you something like what it already knows.”
I want to introduce you to a new term. At Deep Space, we have a proprietary data framework that we call Core Thread Technology. Part of that technology framework is Embodied Intelligence. Let’s define it..
Embodied Intelligence is found when a computerised system has inbuilt comprehension of data classification, relationships, workflows, and qualitative measures. This inbuilt comprehension is encoded into the system by humans based on a depth of real world experiences, probably over decades.
Does Deep Space have AGI right now? No.
But Deep Space does have Embodied Intelligence.
We have already established that the majority of cool and powerful AI tech currently available has been trained on raw data originally and usually attributed to human agents. I believe the AI term itself is really too generous for the majority of the currently available tech. But Embodied Intelligence is a practical term that embraces the fact that there are experienced industry leaders who can legitimately train a system in specific ways to maximise productivity.
That is what we are doing for Digital Design and Construction at Deep Space right now.
Is anyone still here?
Yes!! And it isn’t an AI bot either! I know it has been a bit quiet around What Revit Wants lately, and you might have guessed that it has something to do with a little startup called Deep Space…
I hope to one day share the whole story from Architectural drafting, to Revit, to Virtual Built Technology (BIM and VDC Consulting), to Revizto, and finally to where we are today – Deep Space!
For now, I just wanted to reconnect and get you thinking…
- What will 2024 hold for our industry?
- Where will the AI stuff finally land?
- Will the global economy stabilise and accelerate?
What Revit Wants has always been about sharing. Sharing insight. Sharing best practice. Sharing the best tools. Sharing the best and craziest hacks to get your job done. And that’s not going to change.
But we are going to talk more about data. About automation. About AI – both real and imagined. About changing things for the better. About breaking down barriers. Barriers that divide us. Barriers in technology. Barriers in communication. Philosophical barriers. Our own self enforced mental barriers!
When I started using Revit, I knew it would be transformative. It was a smarter way to work! When we discovered Revizto, I was glad someone had built the tool I had sketched and prototyped in 2014 – an integrated collaboration platform for 2D, 3D and more. As we built Deep Space, we knew we were entering a new generation – The Next Generation. Something that would be practical, data-focused, agnostic, embodied with years of domain expertise. We called our proprietary engine “Core Thread Technology”.
Anybody can build dashboards, but only Deep Space has a data-first, structured, relational, historical, self aware, replicated, highly available, predictable, tenanted engine for capturing, storing and automating real digital design and construction workflows at both project and portfolio level…
I spoke to someone recently and we talked about “data, the new gold”. He laughed and said that phrase was used in some industries back in the 90s!! So ‘data’ isn’t really new. But for specific industries, at various times, it gets unearthed and polished and shaped into something valuable. That is happening rapidly now in AEC and Digital Construction. That is what Deep Space was built to do. Mine this AEC golden data, clean it, polish it, connect it with other gems of information and make something both beautiful and useful and transformative. Let’s actually make the most of the data we have!
Let’s use data to build better projects faster, and let’s start right now.
Look out for more posts and updates coming soon…
I’m very excited to report that the Deep Space platform continues to rapidly evolve, bringing exciting updates that empower civil and infrastructure projects. In a recent release, Deep Space introduced new and enhanced existing synchronization plugins and tools, revolutionizing data acquisition, analysis, and compliance checking. This blog post delves into the key new features of the Deep Space Sync add-in, focusing on its IFC integration and LOI (Level of Information) Report capabilities. Jump straight to the video here.
Simplifying Data Acquisition and Storage
Deep Space now offers a user-friendly IFC Sync plugin, enabling users to effortlessly load data from IFC (Industry Foundation Classes) files into the platform. By selecting the desired IFC file, workspace, and project, users can seamlessly upload the information to Deep Space. This streamlined process ensures that different data and model formats, such as IFC and Revit files, are easily accessible and integrated within the platform.
Exploring Data and Parameter Analysis
Once the data is uploaded, users can navigate to the Deep Space Explorer platform to explore various applications. The Data app provides a comprehensive overview of the acquired information from the IFC file. Users can visualize the data, including the element count, parameter summaries, and individual parameter values. By clicking on specific objects, users can access detailed parameter data, facilitating a deeper understanding of the model.
Efficient Compliance Checking with LOI Reports
Deep Space’s LOI Report app is a powerful tool for compliance checking, particularly for projects adhering to government requirements like the Transport for NSW Digital Engineering Standards. The app automates the verification process, comparing the required parameters against the actual data within the IFC and Revit-based models. By unifying both types of data, Deep Space provides a single platform for comprehensive checking, streamlining the compliance process.
Advanced Analysis and Customization
The LOI Report offers an array of functionalities for in-depth analysis. Users can check the existence of parameters and validate the presence of data within those fields. Deep Space also supports the verification of shared parameter GUIDs and duplicates, ensuring the uniqueness of asset IDs. Through drill-down capabilities, users can access specific files, view available information, and explore the requirements tied to each parameter set.
Configuration and Automation
Deep Space provides a robust configuration engine (we call it DS Command), allowing users to modify templates, load parameter standards, and create a master parameter set for consistent use across projects. Additionally, the platform offers scheduling capabilities, enabling users to automate the export of models at predefined times. By simplifying configuration and scheduling tasks, Deep Space optimizes efficiency and reduces manual effort.
Conclusion
The latest Deep Space release showcases its commitment to overcoming industry challenges related to diverse data formats, data acquisition, and analysis. With the IFC Sync add-in and updated LOI Report app, Deep Space provides a comprehensive solution for integrating IFC files, performing compliance checks, and ensuring predictable outcomes. By leveraging Deep Space’s powerful features, Digital Engineers and Design and Construction teams can accelerate project delivery, reduce risks, and enhance overall quality.
I was recently working on a multi-vector dataset comparison in Deep Space. We had received Revit, Navisworks and tabular data, and I was comparing 3 different data drops of that information, particularly for changes in the quantities of specific types of elements. A ‘data drop’ is a set of data or files that you receive at a given point in time.
In the course of exporting the Navisworks data through to CSV, I came across a specific problem – the number of rows in imported CSV did not match the number of elements processed. Why?
As you may know, you can store line breaks inside parameters in Revit. There are very few good reasons to do this, but it still does happen. Once this happens, those line breaks need to be processed by tools down stream. I spent a lot of time in the early days of Deep Space figuring out how to ‘clean’ bad Revit data so we could still bring it into the platform for analysis. Usually, if the CSV writer or reader are smart enough, they should be able to deal with this problem. However, I discovered a bit of a gap in the available tools this time. What was the problem?
It turns out that the CSV was malformed, it was actually dirty or bad data. While it did have the line breaks, it did not consistently use double quotes to contain fields. So we had the situations where there would be line breaks that were inside the CSV fields or columns, but not inside double quotes. I tried a lot of different CSV readers, including Excel, LibreOffice, Google Sheets and PowerBI / PowerQuery, but they all tripped up at this data. Because they were using the rule ‘new line = new row of data’, the imported CSV information was coming in mangled.
How can we clean this data? We generally know there should be 17 fields or 16 commas per row of data. But we also know there can be line breaks inside fields… so it is a challenge to map a data row to CSV lines. In some cases 2 or 3 lines of CSV data might still just be one row of actual data.
After trying to use various out of the box solutions, I decided to build some Python code to try and solve this. I used Dynamo Sandbox 1.3 to do this, primarily out of habit, not because it is the best Python IDE out there 🙂 I ended up with a kind of line-merging iterator, here is some of the Python code below:
biglist = IN[0] commact=[] for ctr in range(len(biglist)): astr=biglist[ctr] strct=astr.count(',') commact.append(strct) counted=range(len(biglist)) fixedstr=[] bad=[] skips=[] incr=0 for ctrx in range(len(biglist)): if ctrx==skips: pass elif commact[ctrx]==16: fixedstr.append(biglist[ctrx]) elif commact[ctrx]>16: fixedstr.append(biglist[ctrx]) elif commact[ctrx]+commact[ctrx+1]==16: jnr=[] jns=biglist[ctrx]+biglist[ctrx+1] fixedstr.append(jns) skips=ctrx+1 else: bad.append(ctrx) OUT = bad, fixedstr, commact, counted
What does it do? Essentially, it counts the number of delimeters (commas) on one line, then it processes or merges lines based on that information.
This actually got me around 90% of the way there. Then I still had to do some manual fixes of things like ‘double double quotes’ that were also tripping up the CSV readers.
Above is a snapshot of the Dynamo script. And here is the script for download:
CSV Line Merge for Malformed CSV
What the the lessons here?
- Try and fix the source or native data if you can. Dealing with messy data downstream can be a real pain.
- If you need to solve this problem, you can pick up my code or work above and advance it a bit further to build a more robust ‘malformed CSV reader’
- Don’t let problems like this distract you during the holidays 🙂
Over the past few years we have been building and refining a data platform for AEC and Infrastructure projects – we call it Deep Space. It has allowed us to manage huge projects, develop powerful analytics, and increase productivity and efficiency with automations.
We just released a brand new version of our Revit addin, check out the first demonstration video here:
Feel free to check out the website at https://www.deepspacesync.com/
We hope to meet you in Deep Space soon!